What we do
Failures are expensive, so we’ve developed preventive maintenance algorithms.
Failures are expensive, so we’ve developed preventive maintenance algorithms that help you look ahead by detecting potential vulnerabilities in large-scale systems and processes, and they can track anomalies that might point to future problems.
Algorithms of this type can estimate the so-called “remaining useful life” of products, assessing how long a machine is likely to run before it needs repair. Such intelligent estimates can bring substantial savings to any business.
Digica has extensive machine learning experience, from standard statistical algorithms to sophisticated deep learning solutions, according to what the project needs. They enable the system to flag up anomalies and assess the remaining life of the product, as well as find reasons for the failure and point to possible solutions.
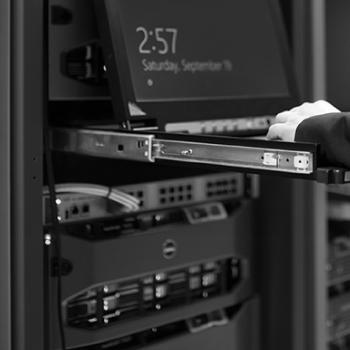